Photorealistic rendering is important for realistic perception of real-world objects which is dependent on the surrounding lighting and surface characteristics of the object. In industry for example in automotive design photorealistic rendering is particularly important and current pipelines for material acquisition involves a lot of cost and time.
Estimating geometry, materials, and lighting from a set of images is often referred to as inverse rendering. Neural radiance fields (NERF) based neural networks directly outputs the color and volume density and hence the radiance is baked in these networks and so cannot be used for relighting. We are working on deep learning based on approaches which can estimate reflectance and lighting for NERF based models. This allows relighting, material editing and more flexible acquisition in NERF based architectures. The challenge here is estimating the reflectance, lighting and geometry with high performance and accuracy and we are actively working in this field to improve the existing the machine learning models.
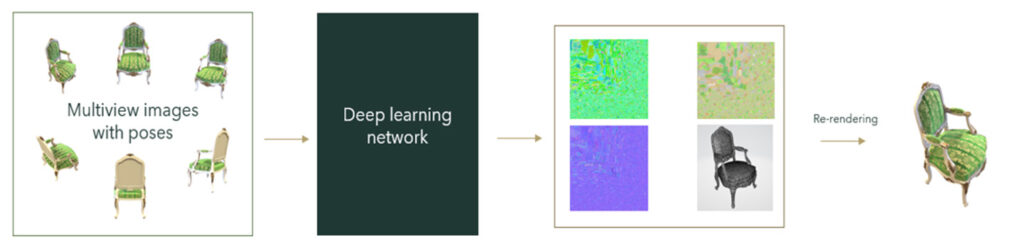
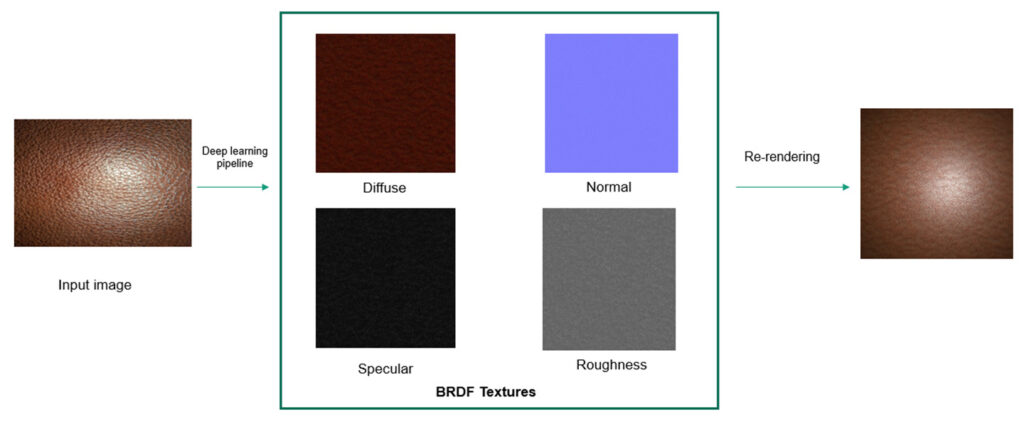
We also developed generative machine learning models for estimating reflectance parameters from single shot flash images for isotropic materials. This approach can help users to get a first impression on how the different flat materials looks on different 3D models and also help designers to speed up the material acquisition process.
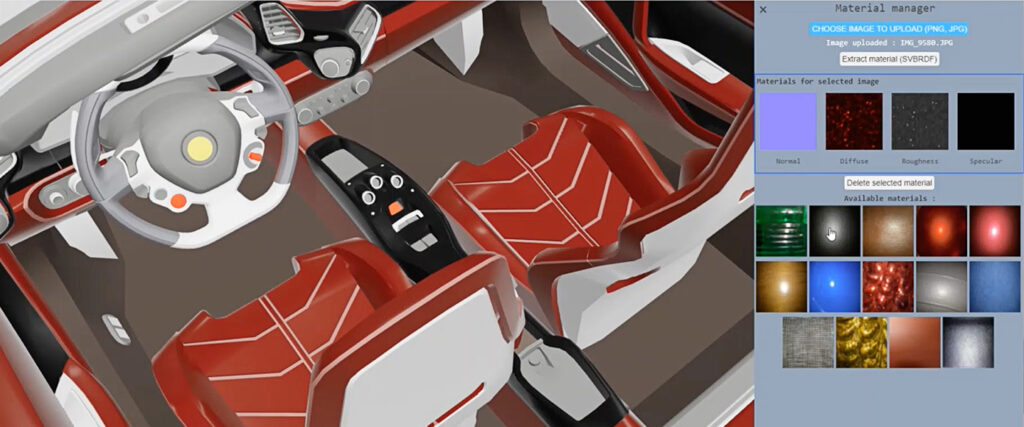